Inside a lab at Google’s headquarters in Mountain View, California, hundreds of server racks are running in multiple corridors, performing tasks far less than those associated with running the world’s main search engine. Instead, these rack servers are conducting tests on Google’s own microchips called Tensor Processing Unit (TPU) are
Google tensor processing units Initially trained for internal workload management, they are available for cloud customers since 2018. Apple announced in July that Tensor processing units for Training AI models which is the foundation of the artificial intelligence platform Apple Intelligence are, uses Google also for training and implementation Gemina chatbot It relies on tensor processing units.
“Daniel Newman”, the CEO of Futurum Group, said this about the competition between Nvidia and Google in the field of AI education:
“There is a fundamental belief around the world that all major AI language models are trained on Nvidia; Undoubtedly, Nvidia is responsible for a large share of the volume of AI education; But Google has also chosen its own path in this area and has been working on them since the launch of Google’s custom cloud chips in 2015.
Google’s position in the field of cloud custom artificial intelligence chip manufacturing
Google was the first cloud service provider to build custom AI chips. Three years later, the first Amazon Web Service Cloud artificial intelligence chip He introduced himself, that is, Inferentia. Microsoft’s first custom artificial intelligence chip, Maia, was not introduced until the end of 2023.
But being the first in the field of artificial intelligence chips means achieving a superior position in the overall competition Generative artificial intelligence has not been Google faced criticism for launching unsuccessful products, and as a result, Gemina was released more than a year after ChatGPT.
However, Google Cloud has gained momentum due to the presentation of its products in the field of AI. Google’s parent company, Alphabet, reported that its cloud revenue rose 29% in the latest quarter, surpassing $10 billion in quarterly revenue for the first time.
Newman said the following about this issue:
“The cloud era of artificial intelligence has completely changed the way companies are seen, and this silicon differentiation (differentiation in the manufacture of chips) or in other words the processing unit itself may be one of the biggest reasons why Google is ranked third due to its artificial intelligence capabilities. The cloud service provider company should achieve a level equal to the level of the other two cloud companies and even a higher position.”
In July, CNBC took the first ever camera-recorded tour of Google’s chip lab and interviewed Amin Vahdat, the head of its custom cloud chip division. He was at the company when Google was first toying with the idea of making chips in 2014.
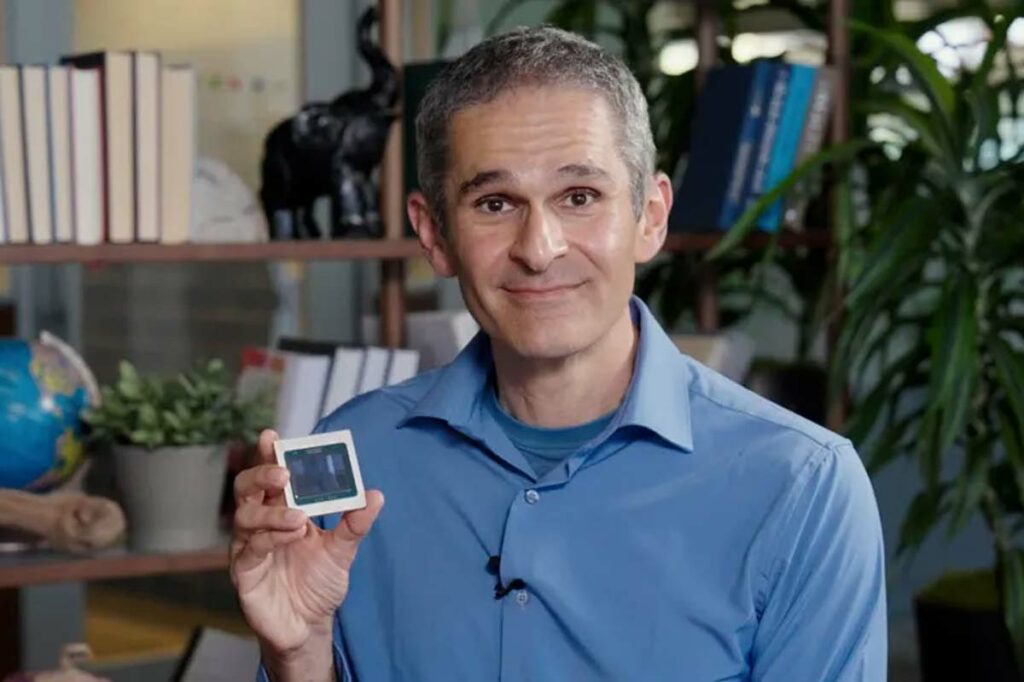
Vahdat said in his interview during the said tour:
“It all started with a simple but powerful thought experiment. A number of company executives asked the question: What would happen if Google users only interacted with Google for 30 seconds a day via voice? How much computing power do we need to support our users?”
At that time, according to experts’ estimates, Google should have doubled the number of computers in its data centers; So they were looking for a basic solution to provide the processing power required by Google.
Vahdat has said this about this issue:
“We found that we could build custom hardware, not generic hardware, but custom hardware (in this case tensor processing units) to support users much better; In fact, 100 times more efficient than support in other conditions.”
Google’s data centers still rely on generic central processing units (CPUs) and Nvidia graphics processing units (GPU) rely on. Google tensor processing units are another type of chip called Program-specific integrated circuit (ASICs) that are customized for specific purposes. TPU focuses on artificial intelligence. Google has also built another video-focused ASIC called the Video Coding Unit (VCU).
Google is also making custom chips for its devices in a similar approach to Apple’s custom silicon strategy. Tensor G4 chip The driving force behind Google’s new Pixel 9 with AI capabilities and the new A1 chip is the driving force behind the Pixel Buds Pro 2.
However, TPU sets Google apart; This processing unit was the first processing unit of its kind released in 2015. Tensor processing units are still the largest among custom cloud AI accelerators with 58% market share, according to a report from FutureChrome Group.
Google coined the term tensor processing unit based on the algebraic term tensor, which refers to the large-scale matrix multiplication that occurs for fast, advanced artificial intelligence applications.
With the release of the second TPU in 2018, Google shifted the focus from inference to training AI models.
Stacey Rosgon, senior semiconductor analyst at Bernstein Research, said:
“GPUs are more programmable and flexible; But their supply has been limited.”
The prosperity of artificial intelligence has caused the value of Nvidia’s stock to increase sharply. The market value of this company reached 3 trillion dollars in June, which was more than the market value of Alphabet. This is while Google was competing with Apple and Microsoft for the position of the most valuable company in the world.
Newman said the following about this issue:
“To be honest, these specialized AI accelerators are not as flexible or powerful as Nvidia’s platform, and that’s what the market is waiting to see: Can anyone compete in this space?”
Now that we know Apple is using Google’s Tensor Processing Units to train its AI models, the real test will be when said AI features are fully rolled out to iPhone and Mac devices next year.
Google cooperation with Broadcom and TSMC
Developing suitable replacements for Nvidia’s AI engines is not an easy task. Google’s sixth generation TPU, called Trillium, is slated to launch later this year.
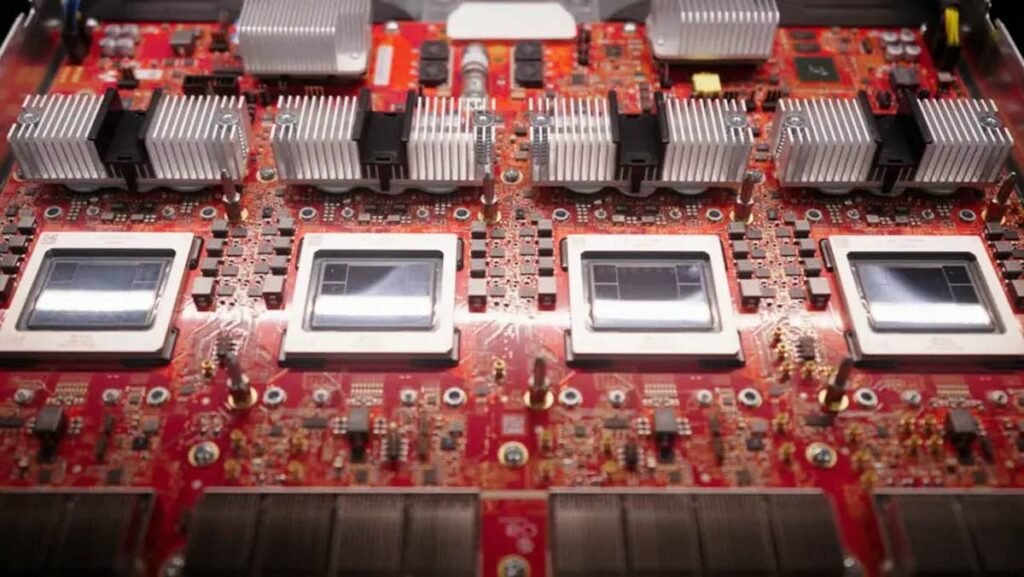
Rasgun said the following about this issue:
“Developing suitable alternatives to AI engines is expensive and difficult; This is not something that everyone can do; But these big data centers have the capabilities, money, and resources to go this route.”
This process is so complex and expensive that even large data centers cannot do it alone. Since the launch of the first TPU, Google has partnered with Broadcom, a chip developer that helps Meta Hamm design AI chips. Bardacom claims to have spent more than 3 billion dollars for these collaborations.
Rasgun has said the following about this matter:
“Broadcom does all the peripherals. This company performs tasks related to receiving inputs and providing outputs, tasks of transmitter-receiver circuits that convert parallel data to serial data and vice versa, and other computing activities. “Broadcom is also responsible for creating protection for the circuit.”
In the next step, the final design is sent to factories for production; These factories are owned by the world’s largest chip maker, TSMC, which produces 92% of the world’s most advanced semiconductor components.
When asked whether Google was considering measures to protect against the worst-case scenario in geopolitics between China and Taiwan, Vahdat said, “We are definitely prepared for such eventualities and we are thinking about them, but we hope that there is no need to act in this case.” It should not be straight.
Protecting against these risks is the main reason why the White House has allocated $52 billion from the Chips Act (CHIPS Act) funds to companies that build chip factories in the United States. To date, Intel, TSMC, and Samsung have received the most funding.
Will Google succeed in its work?
Regardless of all the risks, Google has made another big move in the field of chips and has announced that the company’s first processor for general applications called Axion will be available by the end of the year.
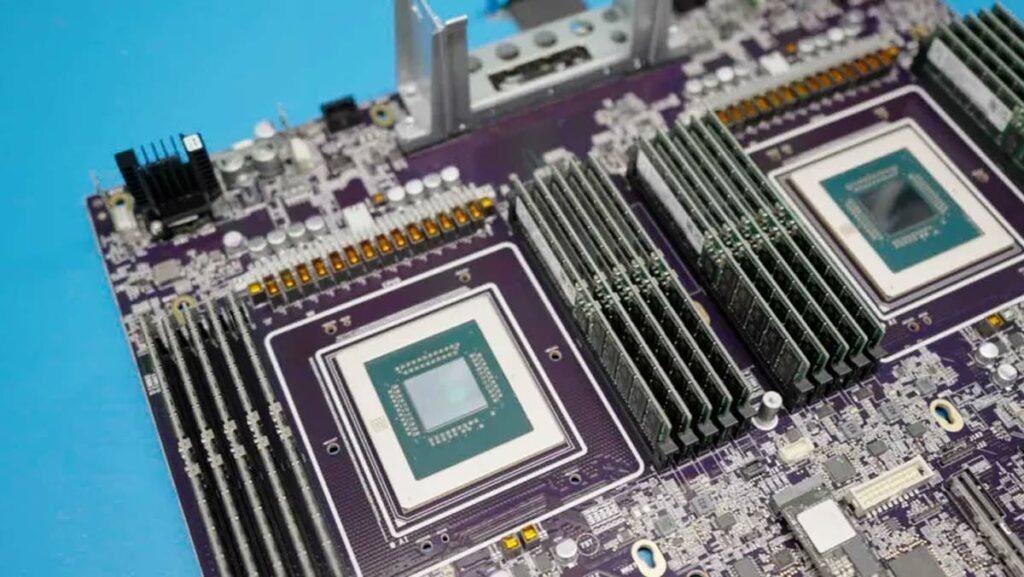
Google is a latecomer to the CPU race. Amazon released its Graviton processor in 2018 and Alibaba released its server chip in 2021. Microsoft also announced its CPU in November.
When Vahdat was asked why Google didn’t start making CPUs earlier, he said:
“Our focus has been on the area where we can provide the most value to our customers, and we’ve started with TPUs, video coding units and networks. “After the release of these hardwares, we believed that the time has come to release the processor.”
All these processors from non-chip companies, including Google, have been made possible by using the ARM chip architecture; The said architecture is a more customizable and more energy-efficient alternative that has been more successful in attracting attention than the traditional x86 architecture used by Intel and AMD. Energy efficiency is very important; Because it is predicted that by 2027, artificial intelligence servers will consume as much electricity as a country like Argentina every year. Google’s recent environmental report found that greenhouse gas emissions increased by nearly 50 percent from 2019 to 2023, in part due to the growth of data centers to power AI.
If the chips designed to use artificial intelligence were not low-power; The mentioned numbers related to environmental damages were much higher than the mentioned amounts; Vahdat has said the following about this issue:
“We are working day and night to reduce the carbon emissions from our infrastructure activities and we are working towards zero emissions.”
Cooling the AI training and execution servers requires a lot of water. For this reason, Google’s third-generation TPU has started using direct-to-chip cooling, which consumes less water. This method, where the coolant moves directly around the chip plate, is the method used by Nvidia to cool Blackwell GPUs.
Despite many challenges, from geopolitics to electricity and water, Google remains committed to delivering productive AI tools and building its own chips.
Vahdat has said this about this issue:
“I’ve never seen Google’s determination, and the company’s momentum has never slowed down, and hardware is going to play a huge role in that.”
RCO NEWS